In recent years, artificial intelligence (AI) has emerged as a powerful force in the transportation and logistics industry, reshaping the way goods are moved from one place to another. While the idea of AI tackling the complexities of logistics might have seemed far-fetched in the past, it’s now proving to be a game-changer.
By harnessing AI technology, logistics companies stand to unlock significant economic value, with estimates suggesting trillions of dollars in potential revenue over the next two decades. Early adopters are already reaping the benefits, enjoying increased profit margins of over 5%.
So, what exactly does AI in Logistics bring to the table, and where does it make the most impact? Let’s explore how AI is transforming logistics, from optimizing warehouse operations to revolutionizing delivery routes with precision timing.
Challenges in Logistics without Artificial Intelligence
The logistics industry faces several challenges that could be significantly alleviated with the adoption of artificial intelligence (AI). However, let’s explore some of the key challenges it faces without AI:
- Inefficient route planning leads to wasted time and resources
- Lack of real-time visibility into inventory and shipments
- Difficulty in predicting demand accurately, leading to overstocking or stockouts
- Manual processes prone to errors and delays
- Limited ability to optimize warehouse operations and space utilization
- Ineffective management of last-mile delivery, resulting in higher costs and customer dissatisfaction
- Inability to leverage data for strategic decision-making and performance improvement
- Higher operational costs due to manual labor and inefficient processes
- Difficulty in adapting to dynamic market conditions and changing customer expectations
- Greater vulnerability to disruptions and supply chain risks due to limited predictive capabilities
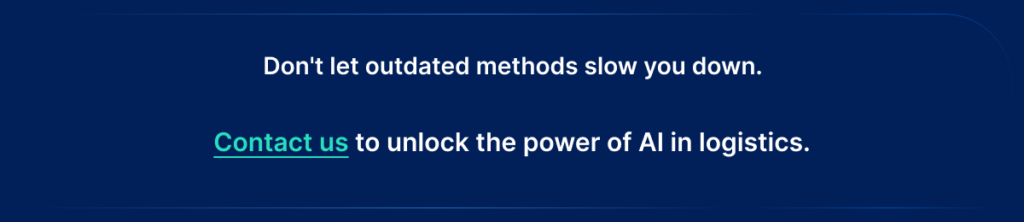
Benefits of Using AI in Logistics Industry
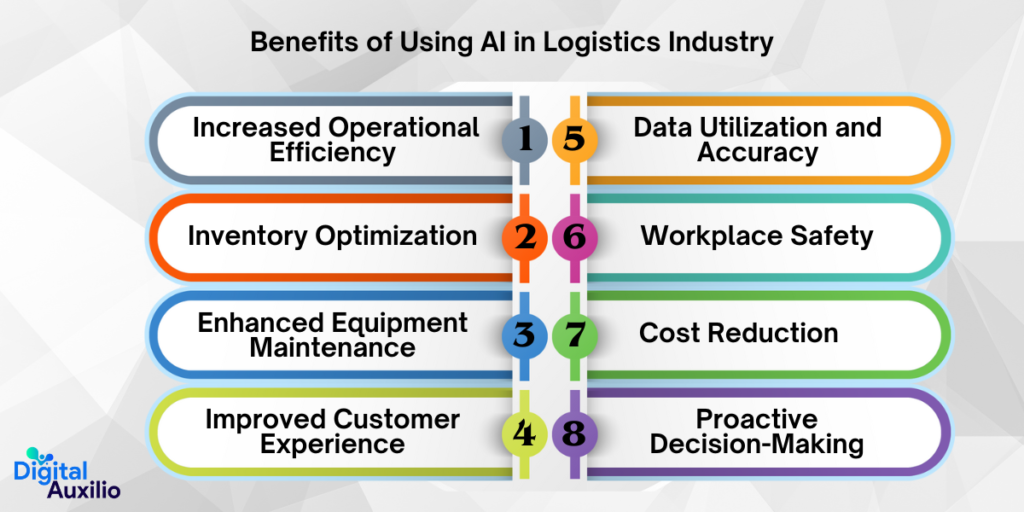
Artificial Intelligence (AI) is revolutionizing the logistics industry, offering a plethora of benefits that streamline operations, enhance efficiency, and improve customer satisfaction.
Here are some key advantages of integrating AI into logistics and supply chain management:
1. Increased Operational Efficiency:
AI-powered systems automate repetitive tasks such as sorting raw materials, demand forecasting, and route planning, reducing manual dependence and optimizing resource utilization. This efficiency leads to faster turnaround times and smoother operations.
2. Inventory Optimization:
AI-driven predictive analytics enable accurate stock forecasting, minimizing overstocking and stock-outs. By analyzing historical data, AI systems maintain optimal inventory levels, reducing storage costs and ensuring product availability, ultimately leading to better customer satisfaction.
3. Enhanced Equipment Maintenance:
AI algorithms coupled with IoT sensors predict equipment failures before they occur, allowing proactive maintenance and minimizing disruptions in supply chain management. This proactive approach saves costs associated with unexpected breakdowns and downtime.
4. Improved Customer Experience:
AI technologies offer various tools such as virtual assistants, real-time notifications, and GPS integrations, enhancing the overall customer experience. From providing delivery schedules to resolving queries promptly, AI-powered solutions ensure customers remain informed and satisfied throughout the logistics process.
5. Data Utilization and Accuracy:
AI capabilities like Natural Language Processing (NLP) and Machine Learning (ML) efficiently gather, organize, and analyze vast amounts of data, improving data accuracy and decision-making. By extracting insights from diverse data sources, AI optimizes shipping processes and resource allocation.
6. Workplace Safety:
AI-based automated technologies contribute to safer warehouse environments by identifying potential hazards and monitoring workplace safety parameters. By analyzing data on safety incidents, AI systems help manufacturers implement preventive measures, ensuring worker and material safety.
7. Cost Reduction:
AI-driven automation reduces reliance on manual efforts, leading to cost savings across various operations, from inventory management to transportation forecasting. By minimizing errors and enhancing productivity, AI systems contribute to operational efficiency and cost-effectiveness in the logistics industry.
8. Proactive Decision-Making:
AI-powered transportation forecasting enables proactive decision-making by predicting market fluctuations and future capacity requirements. By leveraging vast amounts of data, AI algorithms help logistics companies anticipate demand and optimize transportation choices, ultimately improving overall supply chain efficiency.
AI In Logistics – Top Use Cases
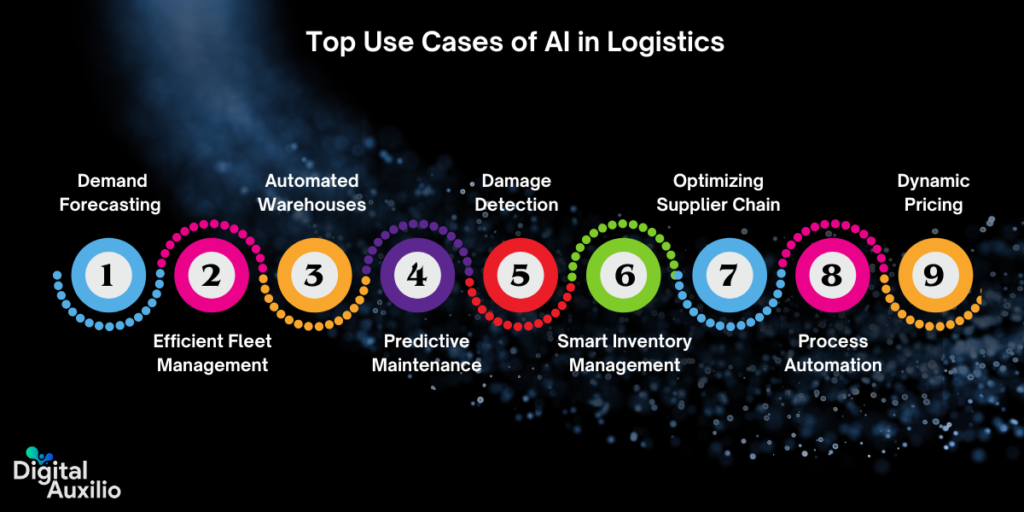
AI has revolutionized the logistics industry, offering innovative solutions to streamline operations, enhance efficiency, and optimize processes. Here are some top use cases of AI in logistics:
1. Demand Forecasting:
- AI plays a crucial role in predicting future demand patterns based on historical data, market trends, seasonal variations, and other relevant factors.
- By employing machine learning algorithms, logistics companies can analyze large datasets to anticipate fluctuations in demand accurately.
- This forecasting enables companies to optimize inventory levels, allocate resources efficiently, and streamline their supply chain operations.
- AI-powered demand forecasting helps reduce stockouts, minimize excess inventory holding costs, and enhance overall customer satisfaction by ensuring products are available when needed.
2. Efficient Fleet Management:
- AI plays a crucial role in optimizing fleet operations by providing real-time insights into vehicle performance, fuel consumption, maintenance needs, and driver behavior.
- Fleet management solutions powered by AI algorithms can optimize the routing, scheduling, and dispatching of vehicles based on factors such as traffic conditions, delivery deadlines, and vehicle capacities.
- Predictive maintenance algorithms analyze vehicle sensor data to identify potential issues before they lead to breakdowns, thereby reducing downtime and maintenance costs.
- AI-enabled telematics systems track vehicle locations, monitor driving behavior, and provide actionable insights to improve driver safety, compliance with regulations, and fuel efficiency.
- Efficient fleet management not only reduces operational costs but also enhances delivery reliability, customer service, and overall competitiveness in the logistics industry.
3. Automated Warehouses:
- AI-driven automation technologies revolutionize warehouse operations by increasing efficiency, accuracy, and speed.
- Automated warehouses leverage technologies such as robotics, IoT sensors, computer vision, and machine learning algorithms to automate various tasks, including picking, packing, sorting, and inventory management.
- Robotics systems powered by AI can handle repetitive tasks, navigate warehouse environments autonomously, and work collaboratively with human workers to fulfill orders quickly and accurately.
- AI-enabled warehouse management systems (WMS) optimize inventory storage, track goods in real-time, and provide valuable insights for inventory replenishment and space utilization.
- Automated warehouses enhance productivity, reduce labor costs, minimize errors, and enable faster order fulfillment, thereby improving overall supply chain performance.
4. Predictive Maintenance:
- Predictive maintenance involves using AI and machine learning algorithms to predict when equipment or vehicles are likely to fail so that maintenance can be performed proactively.
- AI analyzes historical maintenance data, equipment sensor data, and operational parameters to identify patterns indicative of potential failures.
- By detecting early warning signs of equipment degradation or malfunction, predictive maintenance algorithms help prevent unplanned downtime, reduce maintenance costs, and extend the lifespan of assets.
- Predictive maintenance enables logistics companies to schedule maintenance activities during planned downtime, optimize spare parts inventory, and allocate resources efficiently.
- Ultimately, predictive maintenance improves asset reliability, enhances operational efficiency, and ensures smooth logistics operations
5. Damage Detection:
- AI-powered image processing and computer vision algorithms can automatically detect and assess damages to vehicles, packages, or goods during transportation and handling.
- By analyzing images or video feeds from cameras installed in transportation vehicles, warehouses, or checkpoints, AI systems can identify dents, scratches, cracks, or other types of damage.
- Damage detection algorithms can help logistics companies quickly identify and address issues, initiate insurance claims, and prevent further damage to goods or vehicles.
- This proactive approach enhances customer satisfaction, reduces losses due to damaged goods, and improves overall operational efficiency in the logistics process.
6. Smart Inventory Management:
- Smart inventory management utilizes AI and data analytics to optimize inventory levels, minimize stockouts, and maximize inventory turnover.
- AI algorithms analyze historical sales data, demand forecasts, lead times, and seasonality patterns to determine optimal inventory levels for each SKU (stock-keeping unit).
- By dynamically adjusting reorder points and safety stock levels, smart inventory management systems ensure that inventory is available when needed while minimizing excess inventory holding costs.
- Advanced analytics techniques, such as demand sensing and probabilistic forecasting, enable logistics companies to respond quickly to changes in customer demand and market conditions.
- Smart inventory management systems integrate with other supply chain processes, such as procurement, production, and distribution, to ensure end-to-end visibility and alignment of inventory levels with business objectives.
7. Optimizing Supplier Chain:
- AI-driven analytics can analyze supplier data, historical performance, market trends, and other factors to identify opportunities for improving supplier relationships, reducing costs, and mitigating risks.
- Predictive analytics can forecast supplier lead times, delivery performance, and potential disruptions, enabling logistics companies to proactively manage their supply chain and optimize inventory levels.
- Supplier segmentation techniques can categorize suppliers based on their strategic importance, performance metrics, and risk profiles, allowing logistics companies to allocate resources effectively and prioritize supplier development efforts.
- AI-powered supplier collaboration platforms facilitate communication, collaboration, and transparency between logistics companies and their suppliers, streamlining procurement processes, and fostering innovation.
8. Process Automation:
- AI and robotic process automation (RPA) technologies automate repetitive and manual tasks in logistics and supply chain processes, such as order processing, invoicing, document management, and data entry.
- Natural language processing (NLP) algorithms enable intelligent document processing, extracting relevant information from unstructured documents such as invoices, purchase orders, and contracts.
- AI-driven workflow automation tools orchestrate end-to-end supply chain processes, automating decision-making, exception handling, and task allocation based on predefined rules and workflows.
- Robotics and autonomous systems automate physical tasks in warehouses and distribution centers, such as picking, packing, sorting, and palletizing, to improve efficiency, accuracy, and throughput.
- Process automation reduces cycle times, minimizes errors, and frees up human resources to focus on value-added activities, such as strategic planning, customer service, and innovation.
9. Dynamic Pricing:
- Dynamic pricing involves adjusting prices in real-time based on various factors such as demand, supply, market conditions, competitor pricing, and customer behavior.
- AI algorithms analyze large volumes of data to identify patterns, trends, and correlations that can inform dynamic pricing strategies.
- By leveraging machine learning models, logistics companies can predict demand fluctuations and optimize prices to maximize revenue and profitability.
- Dynamic pricing algorithms can be applied across various areas of logistics, including freight transportation, warehousing services, and last-mile delivery.
- By dynamically adjusting prices, logistics companies can capitalize on opportunities, optimize resource allocation, and remain competitive in dynamic market environments.
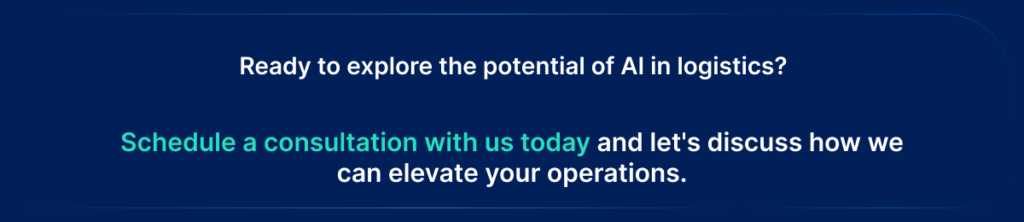
Companies that Use AI in Logistics
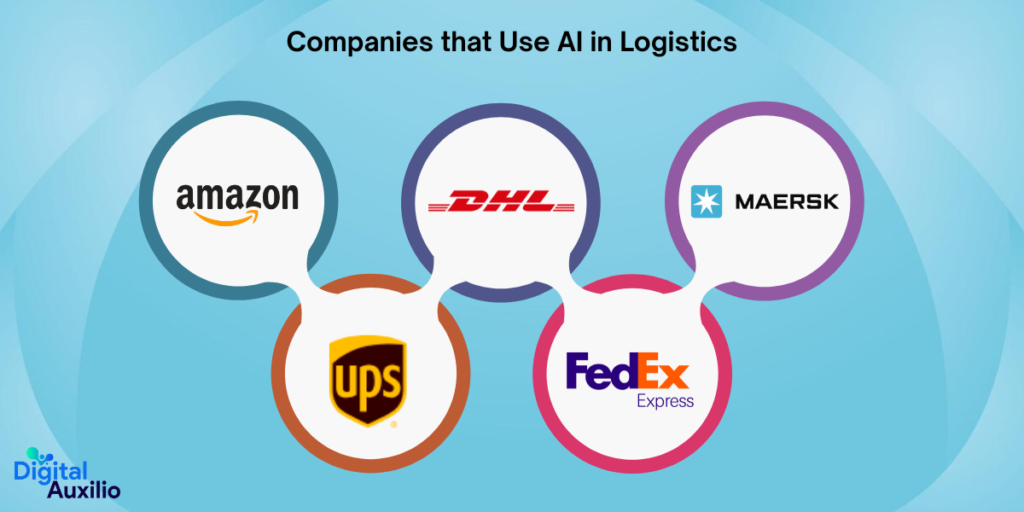
In today’s fast-paced world, logistics companies are increasingly turning to artificial intelligence (AI) to streamline their operations and stay ahead of the curve. From optimizing route planning to enhancing inventory management, AI is transforming the way goods are transported, stored, and delivered.
Let’s explore how leading companies are leveraging AI in logistics to drive efficiency and deliver superior service.
1. Amazon:
As one of the pioneers in AI-driven logistics, Amazon utilizes machine learning algorithms to forecast demand, optimize warehouse operations, and improve delivery routes. Their sophisticated AI systems analyze vast amounts of data in real-time to ensure timely deliveries and minimize costs.
2. UPS:
With its ORION (On-Road Integrated Optimization and Navigation) system, UPS harnesses AI to optimize delivery routes for its fleet of vehicles. By considering factors like traffic patterns, package sizes, and delivery windows, UPS reduces fuel consumption, emissions, and overall delivery time, leading to substantial cost savings.
3. DHL:
DHL employs AI-powered predictive analytics to anticipate supply chain disruptions and mitigate risks proactively. By analyzing historical data and external factors such as weather conditions and geopolitical events, DHL optimizes inventory levels, reduces stockouts, and improves customer satisfaction.
4. FedEx:
FedEx leverages AI and machine learning algorithms to automate sorting processes in its distribution centers. By accurately identifying packages and determining their optimal placement for loading onto delivery trucks, FedEx minimizes errors and expedites the sorting process, enabling faster order fulfillment.
5. Maersk:
As a leader in maritime logistics, Maersk utilizes AI to optimize container shipping routes and vessel scheduling. By analyzing various factors like weather patterns, port congestion, and fuel consumption, Maersk maximizes operational efficiency while reducing carbon emissions and transportation costs.
In Conclusion
AI is revolutionizing logistics by improving efficiency, accuracy, and cost-effectiveness. Skilled AI developers are driving this transformation, helping companies streamline operations, optimize routes, and manage inventory effectively.
If you’re looking to harness AI development services to reshape the logistics, contact us at Digital Auxilio, your trusted AI development company.
FAQs
What are the benefits of using AI in logistics?
Improved operational efficiency through optimized route planning, inventory management, and resource allocation.Enhanced predictive analytics for demand forecasting, reducing stockouts and overstock situations.Real-time tracking and monitoring of shipments, leading to better visibility and responsiveness.Automation of repetitive tasks, freeing up human resources for more strategic activities.Cost reduction through minimized errors, fuel consumption optimization, and streamlined processes.
What are the main challenges associated with implementing AI in logistics?
Data quality and integration: Ensuring accurate and comprehensive data is available and compatible across various systems.Implementation costs: Initial investment in AI infrastructure and technology may be substantial.Talent shortage: There is a shortage of skilled professionals who can develop, implement, and maintain AI solutions in logistics.Regulatory and ethical concerns: Addressing privacy, security, and fairness issues related to AI use in logistics.
How does AI improve last-mile delivery?
AI helps optimize delivery routes in real time, considering factors like traffic, weather, and delivery windows, to ensure timely and efficient last-mile delivery.Machine learning algorithms analyze historical data to predict delivery times more accurately, reducing instances of missed or late deliveries.AI-powered tracking and monitoring systems provide customers with real-time updates on the status of their deliveries, enhancing transparency and customer satisfaction.Automation technologies such as delivery drones and autonomous vehicles are being explored to further streamline last-mile delivery processes.
Add comment